top of page
Home: Bienvenue
Our customers

.png)
.png)
.png)
.png)
.png)
.png)
.png)
.png)
.png)
.png)
.png)
.png)
.png)
.png)
.png)
.png)
.png)
.png)
.png)
.png)
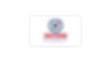.png)
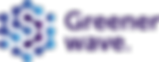
What our clients say

Our partners




.png)






Prizes

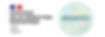
ADAGOS has received the GreenTech Innovation label.
This label, awarded by the Ministry of ecology and solidarity Transition, selects companies that develop ambitious, innovative and sustainable projects.
Press release

ADAGOS wins the CONTINENTAL start-up challenge Grand Prix 2019.
ADAGOS drastically reduces the size of artificial neural networks, enabling Continental to embed them in their cars of the future.
Press release
bottom of page